Kartezio: a fully transparent AI-based approach to biomedical image analysis
Artificial intelligence,
,
image processing,
machine learning,
immuno-oncology,
cytotoxic T cells.
Brienne McKenzie and Salvatore Valitutti DynAct Molecular dynamics of Lymphocyte Interactions
In digital medicine, artificial intelligence (AI) algorithms used for medical image analysis should not only be highly accurate, but also fully transparent and interpretable, in order to build trust, ensure compliance with ethical standards, and facilitate regulatory oversight. Many high-performing Deep Learning-based algorithms are nonetheless inherently difficult to interpret due to their “black box” nature, as well as being computationally expensive and needing huge datasets for training. In this study, we introduce Kartezio, a first-in-class evolutionary AI approach that generates fully transparent and easily interpretable “white box” image analysis algorithms. Kartezio matched the performance of state-of-the-art Deep Learning approaches on specific image processing tasks, while requiring only a handful of images to train. We validated this ground-breaking approach using a panel of case studies in Immuno-Oncology, ranging from delineation of whole tumors in pathology to detection of subcellular particles using super-resolution microscopy. We showed Kartezio to be precise, robust, flexible, fast, and fully transparent to humans, making it a game-changer in AI-based biomedical image analysis.
Our work provides a new fully transparent AI-based approach to medical image analysis, with broad potential applications across clinical disciplines, including pathology and radiology, as well as microscopy. We validated our unique approach in multiple Immuno-Oncology case studies, including the delineation of whole tumors and the detection of cytotoxic subcellular particles. These case studies highlight how impactful this highly accurate, fully transparent AI approach will be in the clinical setting and the diverse range of applications for which it has relevance.
The described approaches can potentially be applied to analyze biomedical images of different source and resolution. It has the potentiality of being used in research, clinical studies and diagnostic.
The following patent application has been filed by KC, SV, SCB, DW, HL, and BM.: “K. Cortacero et al. filed patent; EP 22307041.8”.
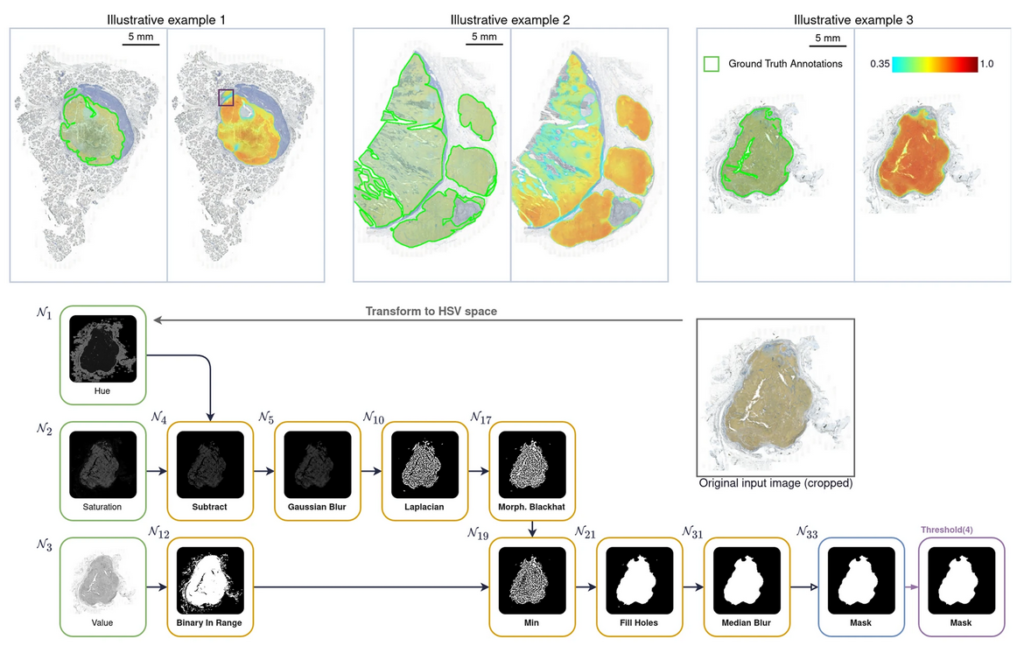
TOP: three examples of melanoma nodules annotated manually by a human expert (green lines) or automatically using Kartezio-generated pipelines (multi-colour heatmap) BOTTOM: an example of a fully transparent, step-by-step image segmentation pipeline generated by Kartezio
Discover the published article
Nat Commun. 2023 Nov 6;14(1):7112.doi: 10.1038/s41467-023-42664-x.
Evolutionary design of explainable algorithms for biomedical image segmentation
Kévin Cortacero, Brienne McKenzie, Sabina Müller, Roxana Khazen, Fanny Lafouresse, Gaëlle Corsaut, Nathalie Van Acker, François-Xavier Frenois, Laurence Lamant, Nicolas Meyer, Béatrice Vergier, Dennis G Wilson, Hervé Luga, Oskar Staufer, Michael L Dustin, Salvatore Valitutti, Sylvain Cussat-Blanc
Collaborations et partenerships
This research stems from the interdisciplinary collaboration between immunologists working at the Cancer research Center of Toulouse (CRCT) and at the Kennedy Institute, University of Oxford (members of the European Research Council funded ATTACK consortium), computer scientists working at the Institute for Research in Informatics of Toulouse (IRIT) and dermatopathologists and dermatoncologists working at Institut Universitaire du Cancer of Toulouse. The work was performed also using HPC resources of the CALMIP Toulouse supercomputing center.
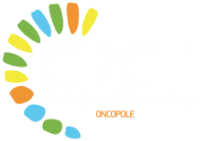
Toulouse Cancer Research Center (Oncopole)
Toulouse - FR
Contact us
+33 5 82 74 15 75
Want to join
the CRCT team ?
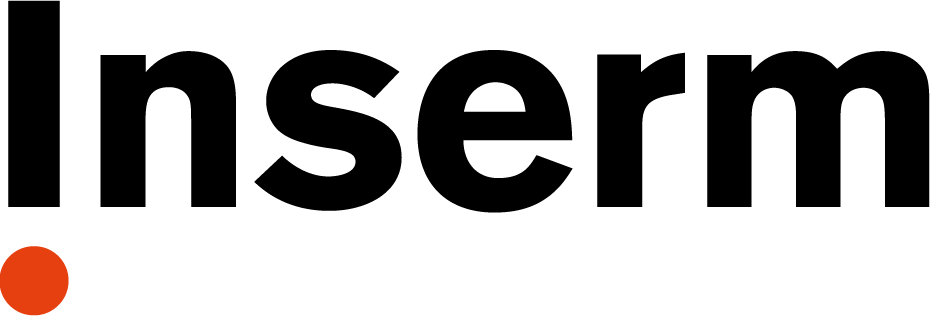
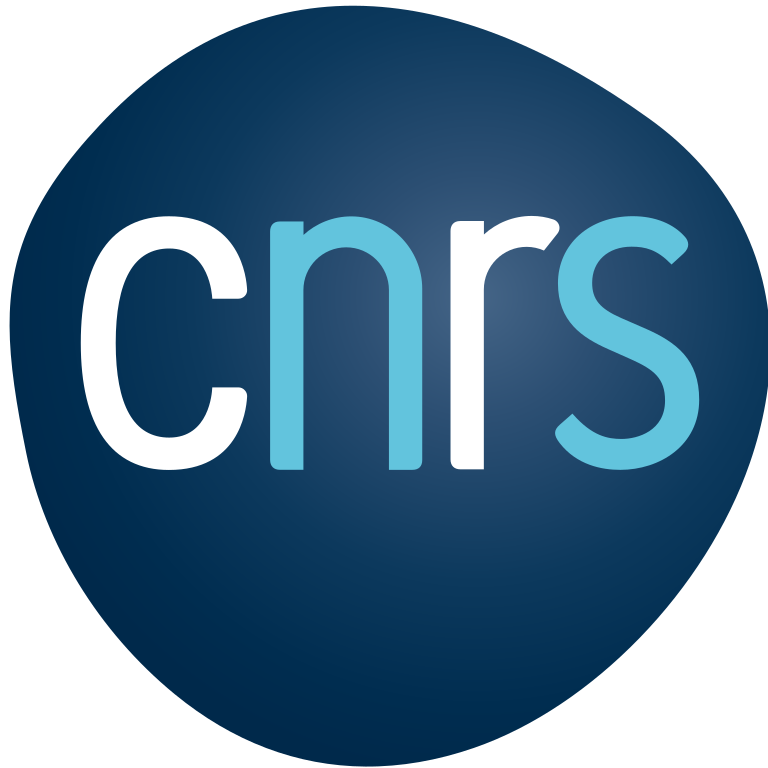
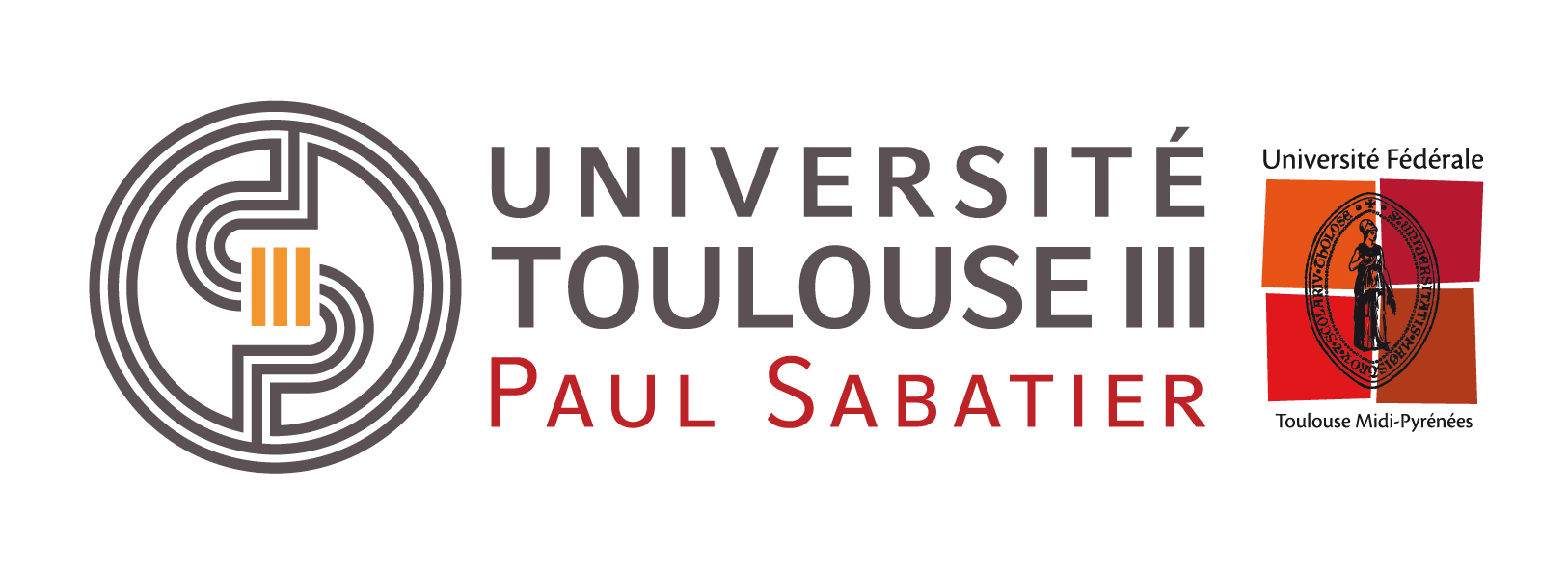